The Practicability of Explainable Artificial Intelligence
- Neelangini Tiwari
- Nov 19, 2023
- 7 min read
Updated: Nov 20, 2023
The author is a former Research Intern at the Indian Society of Artificial Intelligence and Law.
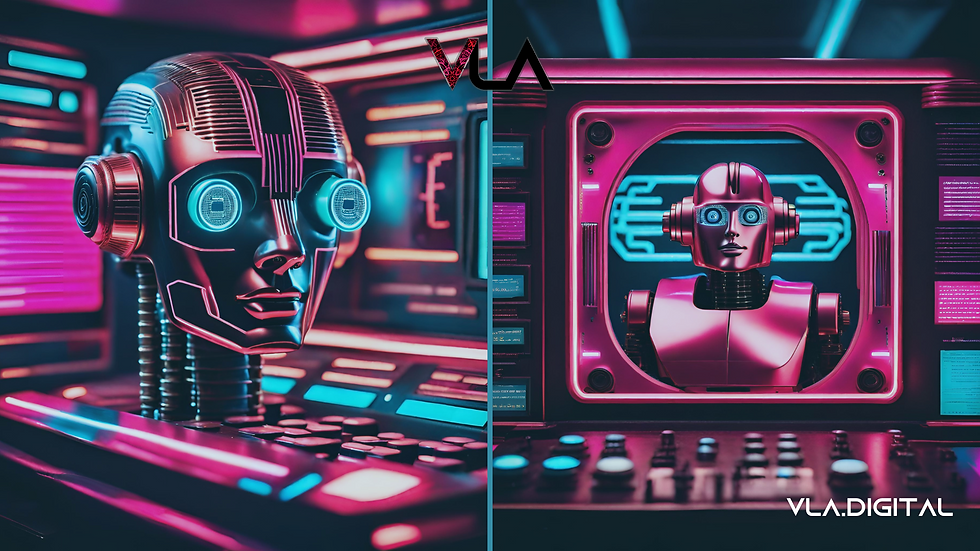
Introduction to XAI
Explainable Artificial Intelligence (XAI) stands at the forefront of the dynamic landscape of artificial intelligence, emphasizing the fundamental principles of transparency and understandability within AI systems. Abbreviated as XAI, this concept embodies a spectrum of methods and techniques utilized in artificial intelligence technology, specifically designed to make the outcomes generated by AI solutions interpretable for human experts. Its primary distinction lies in direct contrast to the opaqueness of "black box" AI systems, renowned for their opaque and inscrutable internal mechanisms.
XAI's core essence revolves around providing a window into the intricate inner workings of AI, primarily focusing on interpretability and predictability. It achieves this by offering various forms of explanations, such as decision rules, white-box models, decision trees, graphs, prototypes, textual explanations, and numerous other methods. Explainable Artificial Intelligence (XAI) operates across diverse tiers of interpretability, each contributing to a deeper understanding of AI systems. At the core is Global Interpretability, focusing on the comprehensive comprehension of an entire model. This level delves into uncovering the fundamental logic that governs the model, shedding light on how input variables intertwine to shape predictions and decisions. In contrast, Local Interpretability zeroes in on individual predictions, seeking to elucidate the rationale behind specific decisions made for distinct instances. Model-Specific Interpretability explores how different types of models function. For instance, it helps in understanding why decision trees are generally more interpretable than neural networks due to their straightforward structure and decision-making process. Lastly, Model-Agnostic Interpretability broadens its scope, offering techniques that explain predictions across diverse machine learning models, irrespective of their complexity or type.
Through these diverse approaches, XAI enables the justification of algorithmic decision-making processes, empowering users to identify, rectify, and exert control over system errors. One of the pivotal strengths of XAI lies in its capacity to uncover learned patterns within AI systems. These revelations not only aid in justifying decisions but also significantly contribute to knowledge discovery within the realm of AI. By unveiling these learned patterns, XAI offers a pathway to comprehending and leveraging the insights gleaned from AI systems, fostering a more informed and empowered approach to utilizing artificial intelligence.
Setting the Context
As AI continues its pervasive integration across diverse societal domains, the legal landscape governing AI regulations is pivoting towards the advocacy of Responsible and Ethical AI. This approach champions principles centred on fairness and transparency within AI systems. However, as the era of autonomous systems unfolds and endeavours towards shaping comprehensive legal frameworks, a conspicuous gap within the Responsible AI approach becomes apparent.
This discrepancy stems from the challenge of imposing a universal ethical standard across all sectors. Diverse functions and varying levels of automation in different AI applications render it impractical to expect, for instance, a large language model engaged in content generation to adhere to the same ethical standards as a medical device performing intricate procedures on humans. The inherent risks, autonomy levels, and degrees of automation vastly differ between these scenarios. Thus, it becomes imperative to comprehend the decision-making processes of autonomous systems and formulate regulations that are not only effective but also tailored to the distinct needs of each domain.
As the proposed Digital India Act of 2023 sets the stage for an impending AI regulatory framework, it becomes crucial to recognize the imperative need for the integration of existing Responsible and Ethical AI principles with Explainable AI. This integration is pivotal in crafting a robust and comprehensive regulatory framework that accounts for transparency, accountability, and domain-specific considerations.
Application of XAI on Different Products
Drug Discovery
The integration of artificial intelligence (AI) and machine learning (ML) technologies has led to a significant transformation in the field of drug discovery. However, as these AI and ML models grow increasingly complex, the demand for transparency and interpretability within these models becomes more pronounced. This necessity has given rise to the emergence of eXplainable Artificial Intelligence (XAI), a novel approach aimed at addressing this specific issue by providing a clearer and more understandable insight into the predictions generated by machine learning models. In recent years, XAI has garnered growing interest and attention, especially concerning its application to the field of drug discovery.
One of the primary advantages of employing XAI in drug discovery is its ability to ensure interpretability. Understanding why a particular compound is predicted to be effective or not is crucial in the realm of drug development, significantly enhancing the efficiency of designing and creating new drugs. Furthermore, as AI and ML models become more intricate, the need for increased transparency is essential, and XAI effectively meets this need by rendering the decision-making processes of these models more transparent. Additionally, the application of XAI in drug discovery extends across various crucial aspects, including target identification, compound design, and toxicity prediction. This broad application highlights the relevance and effectiveness of XAI in multiple stages of the drug development process.
Fraud Detection
Within the domain of fraud detection, one facet of XAI involves employing transparent techniques such as decision trees and Bayesian models. These methods inherently offer interpretability by outlining clear rules that govern their decisions, ultimately making the decision-making processes more understandable for human investigators. Another critical dimension of XAI in fraud detection revolves around making complex models, like neural networks and deep learning algorithms, more "explainable". This pursuit involves the development of specific methods tailored to interpret the decisions made by these intricate models, thereby shedding light on the reasoning behind their predictions.
The explanations provided by XAI in the context of fraud detection play a pivotal role in aiding investigators to discern how and why AI arrived at specific conclusions. For instance, these insights might uncover instances where healthcare providers bill for services not rendered or overbill for services beyond appropriate reimbursement rates. Furthermore, the integration of fraud detection models within the operational workflows of insurance companies optimizes the identification and verification steps, reducing operational costs and increasing processing efficiency. These models efficiently sift through legitimate claims, streamlining the workload for fraud investigators and allowing them to focus on more suspicious cases.
Self-Driving Cars
Explainable AI (XAI) stands as a critical linchpin in the advancement and wider acceptance of autonomous vehicles (AVs) and self-driving cars. Its role is fundamental in rendering AVs more comprehensible, reliable, and socially embraced. Here's how XAI contributes to this transformative process:
Firstly, it fosters trust and transparency by providing clear insights into the decision-making processes of AI-driven autonomous vehicles, crucial for users to understand and trust this advanced technology. Additionally, XAI ensures regulatory compliance, assisting AVs in explaining their decisions to align with the diverse legal requirements across different jurisdictions. XAI's contribution extends further by enhancing both the safety and transparency of autonomous driving technology, garnering support from regulatory bodies and actively engaged stakeholders. This support is pivotal in bolstering public confidence in these technological advancements, which constantly make intricate real-time decisions.
The application of XAI within the realm of Autonomous Vehicles encompasses several key areas. For instance, it is instrumental in explaining semantic segmentation predictions derived from the input frames observed by AVs, aiding in understanding how the vehicle's perception system identifies and categorizes objects, crucial for safe navigation. Moreover, XAI plays a vital role in various dimensions of AV systems, including perception, planning, and control, ensuring an understanding of how the vehicle perceives and manages objects within its environment for safe navigation and operation.
Recommendations
The development and implementation of AI frameworks in India are of critical importance as artificial intelligence becomes increasingly integrated into various aspects of society. Here are comprehensive suggestions to fortify the incorporation of eXplainable AI (XAI) and its ethical application:
Emphasis on Explainability
With AI playing an expanding role in our daily lives, prioritizing the integration of explainability within AI systems becomes paramount. Policymakers should consider mandating explainability in AI regulations, thereby encouraging the development of transparent and easily understandable AI systems. This move will pave the way for greater trust and comprehension among users.
Collaborative Frameworks
Policymakers need to foster collaboration between AI developers, subject matter experts, and policymakers to formulate guidelines specifically tailored for XAI implementation in various sectors. This collaborative endeavour will ensure that XAI is effectively applied while meeting the specific requirements and standards of different domains.
Validation and Training Enhancement
When integrating explainable AI, validating methods and explanations in a user-friendly format is crucial. Shifting focus from merely evaluating explanations to incorporating explanation quality metrics into the training process is essential. This approach ensures that future XAI models are not only accurate but also proficient in providing understandable explanations, enhancing their usability and transparency.
Legal Framework and Policy Integration
The Indian government should consider establishing a comprehensive legal framework governing the deployment of XAI technologies. This framework should encompass regulations overseeing AI applications and effectively address potential hurdles that may arise. Additionally, it is crucial to consciously prioritize the development of 'XAI' and related concepts such as 'Differential Privacy' by implementing methodologies like 'Federated Learning' within policy documentation.
Implementing these recommendations will not only ensure the ethical and responsible deployment of AI technologies but also encourage the integration of transparent and accountable AI systems within India's regulatory framework.
Conclusion
The evolution of Explainable AI (XAI) has marked a pivotal shift in the landscape of artificial intelligence, emphasizing transparency and understandability within AI systems. Its diverse spectrum of interpretability levels, from the global to the specific, provides crucial insights into the decision-making processes of AI, enabling profound understanding and trust. As AI increasingly integrates into society, prioritizing explainability within these systems becomes imperative. Policymakers must mandate the integration of explainability into AI regulations, fostering transparent and easily comprehensible AI systems. Collaborative efforts among developers, experts, and policymakers are essential to tailor guidelines for sector-specific XAI implementation. Validating methods and explanations in a user-friendly format is crucial when integrating XAI, necessitating a shift from evaluating explanations to including explanation quality metrics in the training process. Moreover, a comprehensive legal framework governing the deployment of XAI technologies should be established, encompassing regulations overseeing AI applications and addressing potential hurdles.
Comments